Introduction In this post, we are going to look at some publicly available data to dig deeper into exploratory data analysis and machine learning techniques. We’ll look at some data from Jonathan McDowell’s Catalog site.
This site has data about man-made objects launched into space and all the debris we have created in near earth orbits.
We’ll try to load the file directly from the site. This allows us to get the most recent data from the internet.
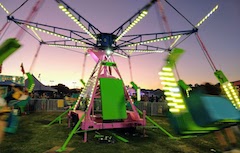